Artificial intelligence (AI) continues to garner considerable interest across the globe. Given the rapid innovations and overbearing presence of AI in professional, commercial, and everyday contexts, acquiring a comprehensive understanding of the potential opportunities and challenges presented by AI is crucial. In the educational sphere, too, attention must be paid to AI to prepare the next generation, which is expected to be at the functional center of an AI-dictated world. To guide this process, AI is accounted for as the 11th grade computer chapter in the US that encompasses both the current trends and the future possibilities to survey the opportunities of exploring a quickly changing field. The significance of educating about AI can be seen in nearly every other sector; for instance, schools prepare students for jobs of the future, scientists and economists predict the trends that could be tackled by AI, while health specialists and clinicians are interested in knowing its pros and cons. This essay tackles some related ideas. It charts the direction of artificial intelligence, identifies many ongoing trends, and makes predictions for the future.
Artificial intelligence is developing at a speed that could make it omnipresent around the world in very little time. It is already a part of several sectors to address a variety of concerns. It also has the potential to shape the world of robotics, allowing machines to learn, adapt, and make decisions. This kind of intelligence enables businesses to grow and saves a lot of time and money; however, ‘intelligent’ machines could outsmart humans and suppress productivity. A broad understanding of the present and likely future of AI would allow necessary personal, business, government, and academic adaptation to ensure productive alignment rather than antagonistic runs or collapses. The insight seeks to unravel what has a grip on AI today before moving on to futurism, which tries to unpack what AI promises to do and how to look for AI to deliver services in the future.
Historical Overview of Artificial Intelligence
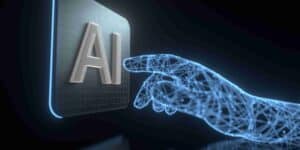
Artificial intelligence today is no longer a philosophical, theoretical concept like it was in the fifties. The ideas of early researchers have long since transcended their purely mathematical evidence and been realized in the form of neural networks, neural modeling, and cybernetics, setting the stage for the first ideas about the learning systems we now recognize as central to artificial intelligence, while also helping to expand the modern idea of the cognitive map beyond its strictly psychological purview. In 1956, a small group of thinkers from two fields—computer science and psychology—gathered to take seriously the possibility of thinking what had previously been unthinkable. Among the central speakers were creators of general models for a variety of systems made of vast numbers of individual, connected parts, and descriptions of cognitive encodings in formal systems filled with symbolic parts.
It was group member John McCarthy, an autodidact, who coined the term artificial intelligence with tongue in cheek for the most whimsical but also prophecy-minded interdisciplinary project of the age. Nearly a dozen conferences, 60 years, and a few “AI winters” later, this utterance is being parsed and teased apart by the world’s most powerful players in fields such as higher education, computer science, robotics, psychology, and quantum mechanics, all of whom continue to boil the problem down. It is being addressed in the form of conferences on AI, and in the special editions of science and technology periodicals, but also in what could be called broad scope policy journals, where issues of neural ethics, the legal standing of robots, and the rights of digital citizens are being determined before the relevant evidence has been adduced to the satisfaction of the court.
Current Trends in Artificial Intelligence
Artificial Intelligence (AI) technologies have been evolving rapidly in recent years, breaking previous records both in terms of accuracy and human-level performance. There are a number of trends that currently dominate the field of AI, and they all share the goal of developing machines that can perform tasks that require human-like intelligence. It is worth noting that the field of AI involves a broad and diverse range of topics, and the word “trends” is frequently used to discuss just a fraction of the field as a whole. The most important trends in artificial intelligence today generally fall under one of four broad subfields: machine learning (ML), deep learning (DL), natural language processing (NLP), and computer vision (CV).
Machine Learning (ML) ML is a subfield of AI specializing in methods and techniques enabling machines to improve at performing a task (or set of tasks) with experience, using data. A significant machine learning trend is the improvement and growing prevalence of machine learning models in predictive analytics and decision-making. Increasingly, companies use machine learning to automate aspects of their business processes and to deploy lightweight, interpretable model/algorithm design for various production systems to be machine learning-ready.
Deep Learning (DL) Deep learning is a subfield of machine learning that focuses on learning methods and algorithms that allow us to solve a wide variety of tasks directly from visual and auditory sensor data. A heavy trend in deep learning today is the design and optimization of more advanced, end-to-end models that outperform previous systems on complex tasks referred to as ‘end-to-end’ learning. These models can perform tasks such as recognizing objects in images, transcribing speech in auditory recordings, analyzing video images, and predicting the trajectory of each item in a scene, and can even develop more complex systems.
Machine Learning
Ever-advancing machine learning (ML) is a branch of artificial intelligence focused on the development of algorithms and systems that learn from and make decisions based on data. Research on AI has been ongoing for decades; however, ML became popular in the mid-2000s due to the significant growth of data. The fundamental objective and advancements in AI and ML have motivated scientists and industries to have a clearer view and perspective for the present and future of AI and ML technologies. There are three types of machine learning: supervised learning, unsupervised learning, and reinforcement learning. Supervised learning methods are widely used in multiple applications. The main challenge is to choose the precise model that minimizes error and overfitting. Good examples include image classification, cancer classification, spam detection, and face recognition. Objects can be classified into different classes or categories. There is unsupervised learning where no labels are present. The objectives include grouping or clustering similar objects from different classes. Moreover, it is essential to identify the relationship between different variables. Unsupervised learning techniques include clustering algorithms, dimensionality reduction, and association rule learning. The k-means algorithm is used mainly in multiple applications, such as in the marketing world, especially to group customers based on their income, and in the healthcare sector, to group patients based on their medications. Moreover, there is reinforcement learning (RL) which applies optimization techniques to help software agents understand their current situation by enabling them to conduct certain operations. RL is essential in game development, robotics, and recommendation. Besides that, there is a significant impact on countless industries worldwide, such as the automotive, financial, healthcare, and marketing fields. For example, in the finance and banking sector, AI and machine learning technologies can be used for fraud detection, stock market prediction, and management of wealth, and in the healthcare and pharmaceuticals sectors, they can be used for drug discovery, treatment plan development, and personalized medicine. Note that the more data, the more accurate AI and ML can be; hence, when big data are available, a significant impact is expected. Limited data restricts the potential of AI and ML methodologies. In addition to data availability, significant improvement in computational power facilitates the development of AI and ML algorithms. The aspects of interpretability, reliability, accuracy, and repeatability of AI and ML are questionable. This is partly because AI and ML algorithms can capture biases unknown to the designers when trained on unprocessed data. Research is ongoing to address these challenges, especially in order to create algorithms that can inspect, recognize, and eliminate the impression of human life. In general, the prospects for the future of AI and machine learning are broad. They may dramatically change many, if not most, of humanity’s needs: transportation, communication, health care, education, security, and entertainment. These technologies may significantly change the processes of managing natural and societal systems, including the design, production, distribution, and handling of physical goods and services, and may even lead to new scientific scales and methods. With current technologies or innovative forms of modeling and activation, new breakthroughs are expected. Furthermore, there is a high possibility for new types of creative integration with different forms of thinking, environmental and social recommendations, as well as unpredictable ethical considerations. Finally, several barriers to general and specific development may still exist.
Deep Learning
Deep learning, a subfield of machine learning, is concerned with algorithms and techniques inspired by the structure and function of the brain in data processing and abstraction. These methods make use of computational models and learning algorithms for finding many layers of representation in order to perform pattern recognition and discovery automatically. Multiple levels of abstraction in deep learning hierarchical models allow systems to learn very complex functions of the data, which can lead to breakthroughs in many worldwide problems. Although deep learning concepts have been around since the 1940s, the current excitement comes from the much larger amounts of training data and the higher computing power available. In practical terms, deep learning methods typically make use of artificial neural networks to model and make sense of the world.
A high-level deep learning network contains several input layers, output layers, and multiple layers of neurons in between. The more layers there are, the deeper the network, hence the term deep learning. Today, deep learning networks can have tens of layers (or more). A neural network’s layers are where the learning takes place; each layer extracts a different level of representation of the data fed into it based on the information extracted by the previous layers. Various types of deep learning are popular, including convolutional neural networks for image recognition, recurrent neural networks for time series analyses, as well as language models, and long short-term memory networks for NLP. Deep learning has come a long way since it was introduced to the world in the beginning of the 2010s. Highly capable research and industry labs have made a tremendous effort to turn proof of concept into real-world applications and have contributed to the development of novel deep learning architectures and algorithms. Today, deep learning is impacting our daily life through a variety of verticals, spanning from healthcare to entertainment, and emerging areas such as autonomous vehicles and precision agriculture.
Natural Language Processing
Natural Language Processing (NLP) is a subfield of AI that deals with the interaction between human language and computers. In the past, linguistics or rule-based programming were used for applications like information retrieval via search engines. These systems faced the challenge of understanding natural language and the context in which words are used. This section discusses NLP techniques and applications.
NLP involves several steps to convert human language to machine-understandable instructions. These steps include tokenization, parsing, lexicon, and semantic analysis. Recent AI techniques based on deep learning and transformers enable advanced applications in NLP. Currently, chatbots, sentiment analysis, machine translation services, and speech recognition are the key NLP areas with practical applications. One critical sub-area is sentiment analysis, which has applications for social media analysis, news, chatbot training, and feedback on products, policies, or services. Sentiment analysis can be used to assess the mood or overall opinion of a particular service or product.
While language models have revolutionized NLP, they are by no means perfect. There are several challenges and concerns. The most significant challenge is the quantity and quality of data. Language models need a large amount of appropriately annotated data to achieve good results. For chatbots or machine translators to work well, we need large corpora of conversations in the multiple languages of interest. However, building large multilingual conversational corpora is challenging and costly. Data privacy and data sharing are additional challenges for language modeling projects. Language models, for the most part, are data-hungry and can never have enough data. A considerable amount of this data is proprietary and sensitive.
Computer Vision
A major part of artificial intelligence involves enabling computers and systems to interpret and understand visual information from the world. This is what computer vision encompasses. Computer vision systems and models take visual information in the form of images or videos as input and then process them according to the underlying learning algorithms that are being used. They are then trained to carry out a variety of tasks, such as object, text, or action recognition, detection, tracking, segmentation, and more, or even classify what they “see” into subjective categories like “beautiful,” “awesome,” etc. Computer vision was traditionally a sub-discipline of image and video processing, which relied on image processing techniques as a pre-processing step to analyzing images. To enable real-time systems and fast analysis of images, video, and multimedia data, traditional image processing algorithms have been replaced by more efficient ones based on learning.
The advancements in recent years in the application of learning algorithms on image and video data, particularly deep learning, have brought about paradigm shifts in the area of computer vision. Today, models with hundreds of layers can significantly outperform traditional learning algorithms with higher accuracy in image classification. The deeper the model, the better the accuracy. Convolutional neural networks have been the biggest revolution in image classification techniques thanks to their in-built model architecture, which enables learning to happen in a hierarchical manner. Continued research in the area of convolutional neural networks is making it possible to significantly reduce overfitting to training data, a central problem in learning. Convolutional neural networks are able to accomplish computational tasks with relatively little preprocessing of the images, typically things like scale, texture, and the relative positions of objects. Many of the current challenges and opportunities with computer vision are in the realm of convolutional neural networks and other learning architectures, applied at scale to large datasets that have been annotated, tagged, or identified in some manner, often by humans.
Applications of AI in Various Industries
AI-driven technologies are rapidly moving from laboratories and industrial facilities to everyday life. In turn, modern businesses are abundantly embracing AI and ML tools throughout a multitude of processes to optimize operations. The predicted worldwide revenue in the enterprise AI market is expected to reach billion by 2025. The industries leveraging artificial intelligence have become far-reaching and evolving. Their incredible progress, when complemented by cutting-edge smart systems, is disrupting key sectors. In more and more cases, AI-boosted apps and solutions might revolutionize multiple industries over the next years.
In the field of healthcare, AI will allow us to advance diagnostics by identifying common patterns and recognizing data for customized treatment recommendations and predictions. In finance, the technology revolution is most likely to touch upon data analysis, risk assessment, and automation. Namely, AI is about to improve the trading process by using complex algorithms designed to optimize trading and flag threats in real-time. The automotive industry will foster rapid changes in the AI segment. By the volume of AI systems in new and used car units will amount to million. Most often, AI is known for its advanced driver-assistance systems, making a head-turner in recent years. Furthermore, AI-based technologies already play a pivotal role in associated industry sectors as they build machines capable of driving autonomously. In the retail sector, AI is widely used in different contexts—ranging from supply-chain optimization and inventory management to customer service and personalized marketing actions. Large distributors use AI to minimize out-of-stock products and increase demand forecasts in the retail industry. Chatbots are now being employed to help consumers navigate websites, channel requests in the call center, and address regular questions. Additionally, advanced personalization tactics and chatbots deploying AI help consumers optimize their in-store and e-commerce transactions. Well-disguised AI recommendations and promotions act as trusty advisors, primarily when pointing out appealing deals and vetted purchases.
AI adoption has been dynamic across industries due to a variety of proactive market forces and demand drivers, although each field faces unique challenges. Manufacturing and retail, for instance, face barriers including a low advance rate following bottom-line evaluation of AI ROI. Fitness, while equally aware of AI’s potential, shows concerns about adopting AI enveloped by legislation associated with privacy and vocabulary barriers. Improved AI-infused products and assistance achieve a much-needed overall performance in ancillary systems. The broader future will be filled with countless alternatives for more disruptive and extensive implementation.
Healthcare
In the first section of this chapter, we examine the role played by artificial intelligence in the healthcare sector. AI applications allow healthcare professionals to maximize the accuracy of diagnostic procedures, develop more effective treatment pathways, and better monitor patients’ health. This is achieved using AI tools that collate large amounts of data from multiple sources, including patient records, lab results, genomic data, and even imaging, and use machine learning to identify trends and predict outcomes based on the probability of a given event occurring. These events could be associated with an individual patient, such as predicting whether a given patient is likely to develop type 2 diabetes in the next 10 years. Alternatively, they could pertain to a particular group of patients, for example, identifying a trend in clinical data that is suggestive of a nascent COVID-19 cluster in a particular region.
In addition to providing patient care, AI solutions are also increasingly being used for administrative purposes. By freeing up time from routine data evaluation and patient monitoring, AI can improve the operating efficiency of healthcare providers and optimize the use of limited resources, including staff resources. There are two critical ethical aspects of AI in healthcare to consider. First, there is the question of who can access data pertinent to patient care and how such data are kept secure. Second, we need to ensure that any algorithm or other decision-making program employed in clinical decisions is itself free from bias. At precisely the time when we are starting to see real-world, practical breakthroughs in AI and healthcare, potential patients are rightly asking whether the use of AI in healthcare really can be trusted. There is a pressing need, therefore, to analyze these tools critically and objectively. Meanwhile, the dialogue between real-world practitioners and those undertaking empirical research is still ongoing, with new approaches bringing further challenges and opportunities.
Finance
The artificial intelligence represents a significant innovation in banking, including innovative customer service, fraud detection, credit scoring, anti-money laundering, and automation of response letters, significantly improving process efficiency to better meet customer needs and provide personalized services. From the technical point of view, electronic advisers are financial experts who use programming logic. This is the derivative of the Robo-Adviser engines—automatic generation of business advice in a somewhat limited area, and this concept has been further extended where business partners offer a decision-support system based on the database and domain expert knowledge, but application expertise is sometimes limited. AI technology and online technologies are expected to increase the quality and breadth of financial advisory services by blending proven advice and best practices with expert advice and enhanced computer-based decision support. AI technology advisors improve the efficiency of financial advisory services. They are not subject to fatigue, work around the clock, and improve speed, reliability, and accuracy. AI assistants today are subject to the regulations of fraud detection, credit scoring for loans, technology advisory/emergency response, automatic generation of business advice, and customer services. Analyzing unusual activities means that market trends show an increasing use of artificial intelligence in stock transactions, either through automated scripts or Robo-advice. Significant savings are expected by transferring jobs—regarding its operations—to robots in customer service, telemarketing, and back offices. More than a hundred private companies are evaluating speech robots, from personal psychologists and financial advisors to personal chefs. FinTechs do not control substantial financial resources, which represents an important outcome of investment in artificial intelligence. However, in addition to the benefits, some research questions, such as unrestricted access in financial markets and the possibility of abuse or IT security, still require detailed scientific research. The processing of mega-data frames privacy issues. Discussing values and fundamental rights should have similar management. There is an ethical point of view, a prerequisite before the fourth industrial revolution. Some practical ethical issues of AI in finance are discussed.
Automotive
Autonomous driving: As of the year 2022, 8 of the 10 top OEMs were offering some form of Level 2 advanced driver assistance system, while 7 out of the top 10 were expecting to launch vehicles with at least a Level 3 autonomous system within the next 3 years. A main difference between a Level 2 system and a Level 3 system is that in a Level 3 vehicle, the electronic control unit processes camera and/or LiDAR signals in real time to make decisions like initiating a lane change or taking over the vehicle, a technology called sensor fusion. In contrast, a Level 2 vehicle requires real-time data to be fused with a detailed map, meaning that the road and its surroundings are already known to the vehicle; thus, intuitive decision-making can take place at a much faster pace. Naturally, a Level 3 autonomous driving system is significantly more complex, with a likely longer and much stricter safety requirement and testing process, as it does not require driver attention under certain conditions.
AI has the potential to completely revolutionize the traffic industry. AI is being used to analyze sensor data from existing traffic and predict future traffic. This information will flow into optimizing the navigation of autonomous vehicles and distribution trucks. Today’s navigation systems optimize the use of road infrastructure, relying on traffic information from other road users. Operators implement traffic lights, and maybe a limited number of sensors observe the traffic. In an automated world, the complete roadway will be observed. Traffic lights, parking space availability, and even road construction will be dynamically optimized to ensure that traffic is flowing, i.e., to prevent breakdown of the system. With better optimization, the addition of any extra infrastructure will be postponed, which decreases costs of a logistics chain and supports the level of autonomy.
Retail
In the retail industry, AI-powered product recommendation algorithms analyze customer behavior to learn preference patterns for product targeting, cross-selling, and upselling. These product recommendations lead to a more personalized customer experience and increase retailer revenue. In the same vein, targeted marketing strategies increase advertising efficiency through process automation and campaign optimization, leveraging customer segmentation to improve relevance to the end user. Inventory management is optimized using AI-powered analytics that forecast product demand. AI solutions are further applied to the supply chain for demand-driven replenishment and in-transit inventory visibility. Furthermore, chatbots help in increasing the level of customer service by answering frequently asked questions. This, combined with the increasing application of virtual assistants, results in the reallocation of human staff to tasks requiring emotional intelligence. Conversely, technological advancements in the industry introduce several ethical considerations. Causes for concern include an overreliance on automated systems, which consume and analyze large volumes of sensitive consumer data, risking breaches of privacy. Furthermore, algorithms have the potential for bias, facilitating unintended discrimination in marketing practices, ultimately affecting brand perception. Consumers are also advised to look for AI solutions with explainable machine learning algorithms in order to ensure companies are making consumer-based decisions in real time. A rapidly growing trend in the industry is augmented reality in retail, using the tool to enhance the retail experience in-store and further personalize the marketing experience. AI technology continues to evolve, with computer vision, natural language processing, interpretable machine learning, and federated AI anticipated to further revolutionize the industry. Success within the sector is contingent on companies innovating while employing their AI tool responsibly. This entails embracing complete transparency, especially in relation to data sharing, and providing human oversight of AI applications to minimize error and maintain customer trust.
Ethical Considerations and Societal Impacts of AI
Business leaders, content developers, product developers, investors, and anyone who uses AI must be mindful of the ethical considerations that bear upon AI. Core to the responsible development and use of AI are values of fairness, accountability, privacy, safety, transparency, non-discrimination, and efficacy, and the continual monitoring of whether and how these values are being realized in practice. The human rights dimensions of AI are multiple. At present, crucial discussions are underway concerning questions of data, data privacy, and the right to explanation of AI-based decisions, in the context of rights to equality and non-discrimination. Advanced uses in labor relations, digital government, policing, and counter-terrorism have raised fundamental questions concerning the potential to exacerbate or cover up societal inequalities and to infringe on rights to free speech and association.
Too little attention to these issues could result in the gradual or sudden erosion of trust in AI, loss of market opportunities, and more generally could fuel broader societal concerns about automation and digital competition. It will be impossible to paper over the societal cracks with positive messaging and public relations. If nothing else, it ought to be in the interests of investors and business leaders to focus on these socially responsible aspects of AI investments. Ethical management of AI will also have other instrumental positive impacts. The considerable investment in automation, using AI, machine learning, and robotics in the workplace has the potential to disrupt working lives and free time so profoundly as to alter the character of human societies in unpredictable ways. In line with previous industrial revolutions, there may be a need to reskill workers and reinvent work and job opportunities in response to automation and job displacement. Cross-government, multi-party, and global initiatives and partnerships are key to maintaining adequate levels of pace in investment and in momentum across public, private, and civil society sectors. In the case of AI and related technologies, they are needed for responsible research and innovation.
Predictions for the Future of AI
Overall, while there is no guaranteed path for the future of AI, there are several specific trends and insights we can take away from the possible avenues for the future of AI. Specifically, we will see more automation across the board, from individual consumer products to entire applications reconfiguring themselves in response to environmental conditions, and more personalization and diversity in AI technologies and workflows, in large part because the initial returns on systems that are developed using generic population-level data and samples have been exhausted. We will see human-AI collaboration expand from mostly developer tooling to more end-user applications and workflows, although truly useful end-user collaboration will demand even more progress on explainable AI. We will see even greater concern and evolution in overcoming AI’s trust gap by providing more of the sorts of transparency features we’ve talked about, especially in the areas of work. International maneuvers toward setting public policy and regulations will take a more comprehensive and inclusive approach to incorporating the public interest in the development of AI. We predict discussions on how superintelligence, in particular, would be regulated and treated, and possible regulation of the creation and usage of artificial general intelligence and of artificial consciousness as well. In the next 1-3 years, we will see more conferences and workshops with broad participation spanning both AI developers and policymakers, and we will move from the collection of principles to the creation of a flag under which groups working on AI and ethics, social justice, and law can coalesce. Moreover, the social impact of AI has now become impossible to ignore, with broad conversations about the future of work, industry, global politics, and humanity itself taking place.